Last week I was lucky enough to attend the Google Impact AI Summit that shared the learning and case studies from Google AI Challenge winners. The Summit included presentations, panel discussions, and a “science fair” where we could see demos from and talk with the winners.
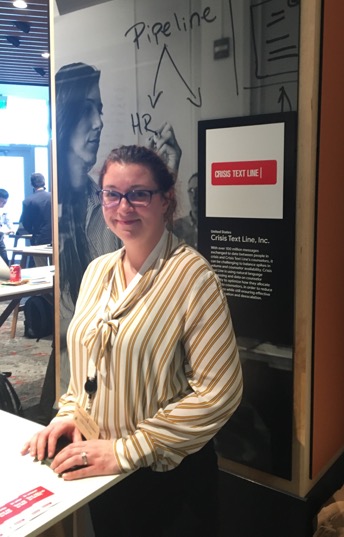
The Summit kicked off with an overview from a report on what they learned about the evolving field of AI4Social Good. The message was clear: Dig into the potential of AI to solve some of the world’s pressing problems.
The panel discussions were focused on Responsible AI, Human-Centered AI, and Scaling Innovation. I happy to see that the Responsible AI panel was all women and included my long-time colleague, Nancy Lublin from CrisisTxt Line one of the AI Impact Challenge recipients. Nancy comments are always right on. She noted that you want your data to be representative of real people.
She went on to say less predictable: “People think that social change organizations should go slow and carefully. They say,move fast and break things should not be applied to social change. Well, screw that. We should move the fastest. We deserve the best people and tech. Our work is about saving lives, not for getting Chinese food delivered at 2 am.”
Google’s challenge was not about cold hard cash, although they did award sizable grants and funding. More importantly it was about innovation and partnerships between those who are close to the problems and techies. The panel session about innovation shared lessons learned from both Google employees who were working with projects as well the nonprofits. Clearly, the challenge was more than just the technology.
There was also a fantastic panel discussion on the way to apply Human-Centered design to AI projects and its importance. The panel was facilitated by Di Dang, Google Developer Design Advocate. I learned about the People + AI Guidebook,
The guidebook focuses on “participatory machine learning,” which actively involves a diversity of stakeholders – technologists, UXers, policymakers, end users, and citizens.” The guidebook provides an overview of how human perception drives every facet of machine learning and offers up worksheets on how to get input.
The guide book stresses the importance of aligning your AI project with users needs. Talking to users, looking through data, and observing behaviors helps shift from technology-first to users-first.
The right questions to be asking:
- Which user problems is AL uniquely positioned to solve?
- How can we augment human capabilities in addition to automating tasks?
- How can we ensure our reward function optimizes AI for the right thing?
Leave a Reply